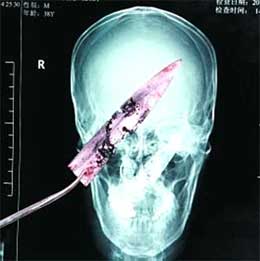
So we get some mild form of source determinism out of Algorithmic Information Complexity (AIC), but we haven’t addressed the form of free will that deals with moral culpability at all. That free will requires that we, as moral agents, are capable of making choices that have moral consequences. Another way of saying it is that given the same circumstances we could have done otherwise. After all, all we have is a series of if/then statements that must be implemented in wetware and they still respond to known stimuli in deterministic ways. Just responding in model-predictable ways to new stimuli doesn’t amount directly to making choices.
Let’s expand the problem a bit, however. Instead of a lock-and-key recognition of integer “foodstuffs” we have uncertain patterns of foodstuffs and fallible recognition systems. Suddenly we have a probability problem with P(food|n) [or even P(food|q(n)) where q is some perception function] governed by Bayesian statistics. Clearly we expect evolution to optimize towards better models, though we know that all kinds of historical and physical contingencies may derail perfect optimization. Still, if we did have perfect optimization, we know what that would look like for certain types of statistical patterns.
What is an optimal induction machine? AIC and variants have been used to define that machine. First, we have Solomonoff induction from around 1960. But we also have Jorma Rissanen’s Minimum Description Length (MDL) theory from 1978 that casts the problem more in terms of continuous distributions. Variants are available, too, from Minimum Message Length, to Akaike’s Information Criterion (AIC, confusingly again), Bayesian Information Criterion (BIC), and on to Structural Risk Minimization via Vapnik-Chervonenkis learning theory.
All of these theories involve some kind of trade-off between model parameters, the relative complexity of model parameters, and the success of the model on the trained exemplars.… Read the rest