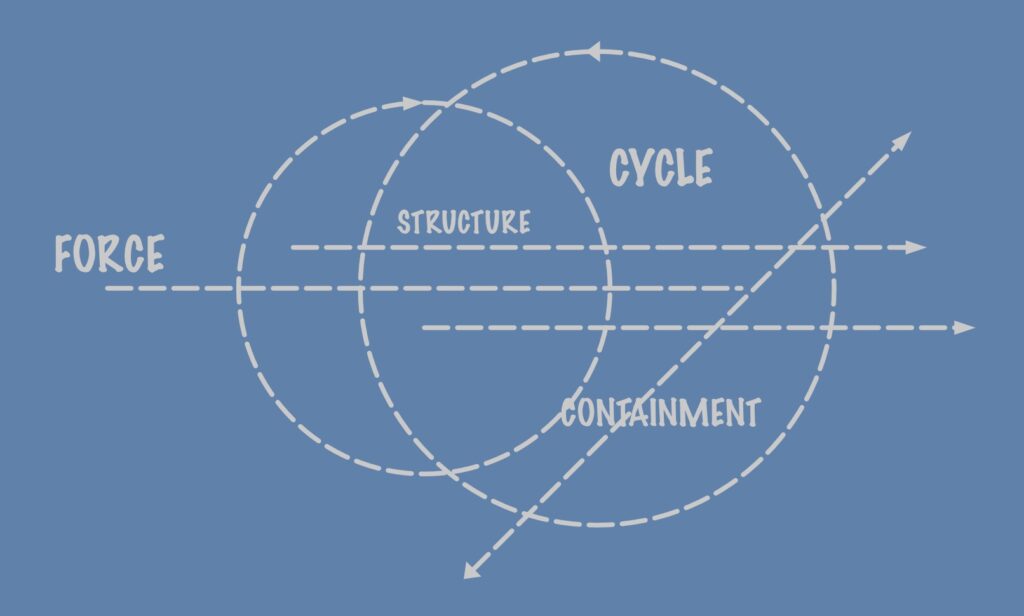
Having recently moved to downtown Portland within spitting distance of Powell’s Books, I had to wander through the bookstore despite my preference for digital books these days. Digital books are easily transported, can be instantly purchased, and can be effortlessly carried in bulk. More, apps like Kindle Reader synchronize across platforms allowing me to read wherever and whenever I want without interruption. But is there a discovery feature to the shopping experience that is missing in the digital universe? I had to find out and hit the poetry and Western Philosophy sections at Powell’s as an experiment. And I did end up with new discoveries that I took home in physical form (I see it as rude to shop brick-and-mortar and then order via Amazon/Kindle), including a Borges poetry compilation and an unexpected little volume, The Body in the Mind, from 1987 by the then-head of University of Oregon’s philosophy department, Mark Johnson.
A physical book seemed apropos of the topic of the second book that focuses on the role of our physical bodies and experiences as central to the construction of meaning. Did our physical evolution and the associated requirements for survival also translate into a shaping of how our minds work? Psychologists and biologists would be surprised that there is any puzzlement over this likelihood, but Johnson is working against the backdrop of analytical philosophy that puts propositional structure as the backbone of linguistic productions and the reasoning that drives them. Mind is disconnected from body in this tradition, and subjects like metaphors are often considered “noncognitive,” which is the negation of something like “reasoned through propositional logic.”
But how do we convert these varied metaphorical concepts derived from physicality into something structured that we can reason about using effective procedures? The technique is based on what Johnson terms image schemata. These schemata are abstract maps that show the generalities that are lurking behind the metaphor. For instance, we know from our physical engagement with the world what a “path” is, although even that can be either very concrete or more abstract. Yet we use an image schemata for paths as a metaphorical substrate for everything from connected narratives that lead us from one state to another, to ideas like our learning of a new topic. Similarly, in Johnson’s semantic theory we have physically-derived image schemata like CONTAINER, CYCLE, LINK, BALANCE, STRUCTURE, FORCE, and others that relate to our evolution and growth as physical beings. When we consider the “architecture” or “structure” of a theory, for instance, we are using a STRUCTURE image schemata as a metaphorical underpinning of the meaning of the statement that is more than just the propositional truth of some statement in some possible world. Instead, the meaning is grounded by our experiences as people interacting with structures.
Johnson, in the 1987 volume, did not see these kind of metaphorical and imaginative expansions of image schemata as algorithmic but wanted them to nonetheless be considered part of reason. I see the issue of the algorithmic component of metaphor and image schemata as critical to making headway on the more general topic of how sentience might arise in a machine implementation. Let’s take a metaphorical abstraction from Convolutional Neural Networks (CNNs). In these networks, images are first processed using mathematical “kernels” that transform the original image into a modified form that exposes reduced properties of the image (a feature map). For instance, we can use simple kernels to expose edges of objects in the image, or to eliminate aspects of the background, or to perform contrast enhancement. These kernels are simple because they are just little 4 x 4 blocks of pixel color or intensity levels that we multiply with the image pixels, working our way across the image from top to bottom. In a pre-conceptual sense, these are exposing aspects of underlying schemata that govern the objects in the images. Once we have these feature maps we can further reduce them by downsampling layers that correlate the statistically distinguishing aspects of the maps to pass on to layers that do categorization of the images into, for instance, categories like dog, cat, bear, wolf, person.
Can we extract similar semantic image schemata from linguistic productions? And, if so, how do they reduce to physical schemata that are essential to the Johnson paradigm? We could try to use something like latent semantic analysis or it’s varied cousins working on the raw words of the statements, but this provides little guidance for this physical categorization. However, if we were to establish a database of categories of statements that are metaphorically related to a specific image schemata, like BALANCE, we could train a deep learning network to categorize additional statements based on that training collection. We might be able to bootstrap that collection by clustering the statements with greater importance given to central terminology involved in the schemata, then build out this CNN-like feature map at the front end that is better informed about physical constraints than just raw ingest of huge collections of online text.
Could this result in the kind of imaginative remapping of ideas to fit into these physical metaphors? It would likely appear to do that in the same sense that current deep learning networks generate text that is occasionally sufficiently interesting that it might be mistaken for human-generated. But clearly the next step is to embody the machinery (even if in an artificial world) such that it internalizes the physical constraints all the way down in the networks prior to layers that build on those models to create metaphors using image schemata.