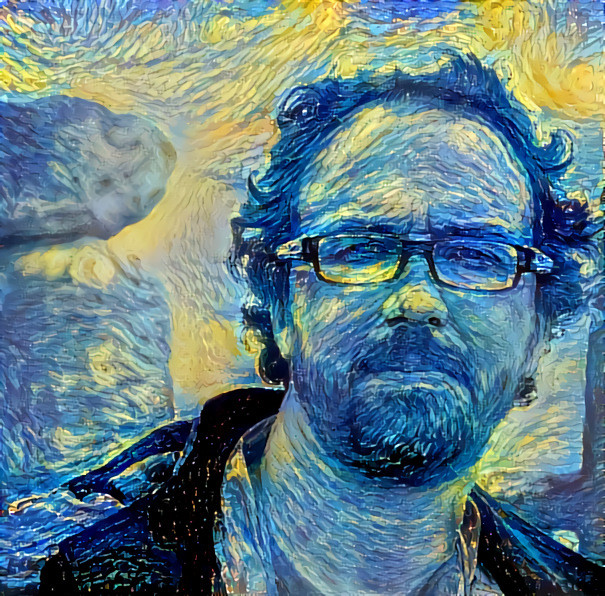
How exactly deep learning models do what they do is at least elusive. Take image recognition as a task. We know that there are decision-making criteria inferred by the hidden layers of the networks. In Convolutional Neural Networks (CNNs), we have further knowledge that locally-receptive fields (or their simulated equivalent) provide a collection of filters that emphasize image features in different ways, from edge detection to rotation-invariant reductions prior to being subjected to a learned categorizer. Yet, the dividing lines between a chair and a small loveseat, or between two faces, is hidden within some non-linear equation composed of these field representations with weights tuned by exemplar presentation.
This elusiveness was at least part of the reason that neural networks and, generally, machine learning-based approaches have had a complicated position in AI research; if you can’t explain how they work, or even fairly characterize their failure modes, maybe we should work harder to understand the support for those decision criteria rather than just build black boxes to execute them?
So when groups use deep learning to produce visual artworks like the recently auctioned work sold by Christie’s for USD 432K, we can be reassured that the murky issue of aesthetics in art appreciation is at least paired with elusiveness in the production machine.
Or is it?
Let’s take Wittgenstein’s ideas about aesthetics as a perhaps slightly murky point of comparison. In Wittgenstein, we are almost always looking at what are effectively games played between and among people. In language, the rules are shared in a culture, a community, and even between individuals. These are semantic limits, dialogue considerations, standardized usages, linguistic pragmatics, expectations, allusions, and much more. The best one can hope to say about the process is that the game rules strive towards coherence much of the time. Aesthetics is similar in that no easily reductive approach can hope to pin down all of the reasons why one piece of art is better than another or a jumble of random associations. (Although that doesn’t mean that there are not other information-theoretic reasons we prefer certain patterns.)
So a non-reductive theory of aesthetics that consists of networks of associations, limits, and congruences, has that same elusive quality of characterization or explication as a deep learning network. For painting, just training a neural network on a history of visual works fails to incorporate all of the determinants that influenced the original artists. Who knows what each had for lunch that day, after all? Or, more to the point, it at best incorporates a secondary or tertiary tier of determinants, already predigested through the assemblage of network influences that converged in the original works. The resulting work is, well, certainly derivative in that derisive sense, but is also lacking in that the influence network is purely perceptual and not cultural.
I’m sure that will change but it does point to the difficulties in even moving from deep learning to general AI, much less human-like intelligence. The systems may need embodiment and cultural immersion and education, and the networks that define these aesthetic games will still remain elusive, much like the how and why of great works of art.