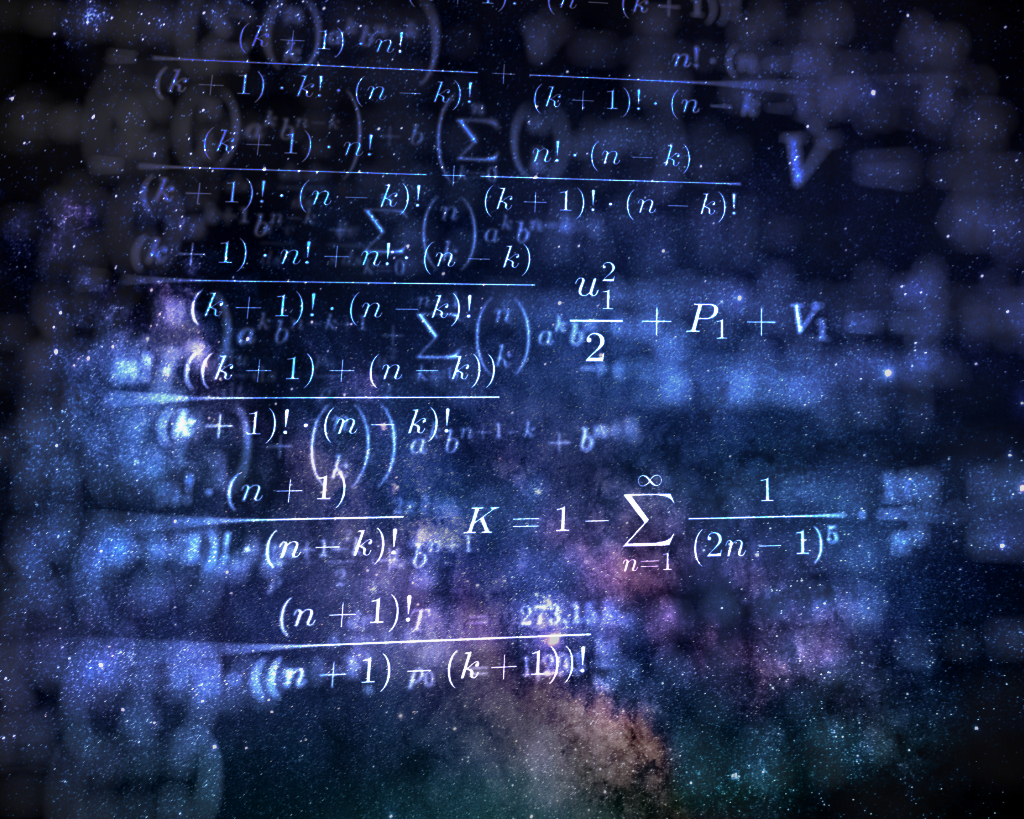
I’ve been enjoying streaming Apple TV+’s Foundation based on Asimov’s classic books. The show is very different from the high-altitude narrative of the books and, frankly, I couldn’t see it being very engaging if it had been rendered the way the books were written. The central premise of predictability of vast civilizations via “psychohistory” always struck me as outlandish, even as a teen. From my limited understanding of actual history it seemed strange that anything that happened in the past fit anything but the roughest of patterns. I nevertheless still read all the “intellectual history” books that come out in the hope that there are underlying veins that explain the surface rocks strewn chaotically across the past. Cousin marriage bans leads to the rise of individualism? Geography is the key? People want to mimic one another? Economic inequality is the actual key?
Each case is built on some theoretical insight that is stabilized by a broad empirical scaffolding. Each sells some books and gets some play in TED and book reviews. But then they seem to pass away out of public awareness because the fad is over and there are always new ideas bubbling up. But maybe that’s because they can’t help us predict the future exactly (well, Piketty perhaps…see below). But what can?
The mysterious world of stocks and bonds is an area where there seems to be no end to speculation (figuratively and literally) about ways to game the system and make money, or even to understand macroeconomic trends. It’s not that economics doesn’t have some empirical powers, it’s just that it still doesn’t have the kind of reliability that we expect from the physical sciences. Here are some examples: Are we heading towards a recession? Were we? Will the S&P rise or fall? And to what level? Is economic inequality significant? Rising? Acceptable?
Around a decade ago there was an effort to use prediction markets to improve on the reliability of predictions like these. A prediction market uses large numbers of people who place bets on the different ideas. The notion is that any one analyst or forecaster might have both an imperfect model and imperfect information. By combining and weighting the predictions a more reliable outcome can be found. Looking carefully at these markets in corporate environments and other deployments shows that they seem to be resistant to manipulation by outsized interests and bets, as well as mildly more reliable than, say, sending out a survey to economists and asking them to assess whether a recession is more or less likely, for instance. There are some caveats, though, like the question needs to be carefully bounded rather than wide open: Is a recession likely in the next six months. There is also some gambling-related psychology like “favorite-longshot bias” that need to be considered.
But prediction markets of this sort are just black boxes. Why is there a consensus that a recession is likely? What are the weights that the participants are attaching to the different underlying indicators and why? In other words, do prediction markets teach us anything about the system and, if they do, can we improve our own modeling as a result?
Here’s one approach: for very constrained questions, we could create a modified prediction market with a requirement that the drivers for the prediction are added in. For example, if I’m using a multivariate linear regression that combines aspects of key economic metrics, and if the model becomes successful in a prediction market, the next step is to look at why that model works. So code up the input data and the model and then, when it wins the market for an econometric prediction, analyze the relative value of the inputs and weights of the model to achieve the winning outcome. For a linear system, the input choice and the weights are what matters and the pile on is based on a belief that the prediction largely aligns with other guesses, sentiments, and gut feelings about the same pool of input data. For more exotic models with nonlinearities and whatnot, the inputs still matter but some kind of spectral analysis might be needed to figure out how the model achieved the results it did (and, frankly, why there was such strange internal amplification of the inputs and what that means). The latter is likely only relevant for weird machine learning methods at the fringes (deep learning, anyone?).
This seems like a logical extension to the core idea of prediction markets as a tool and may help to bridge from model to something more like what Hari Seldon and Isaac Asimov envisioned.
Edits! Hari Seldon!